What is a data lake?
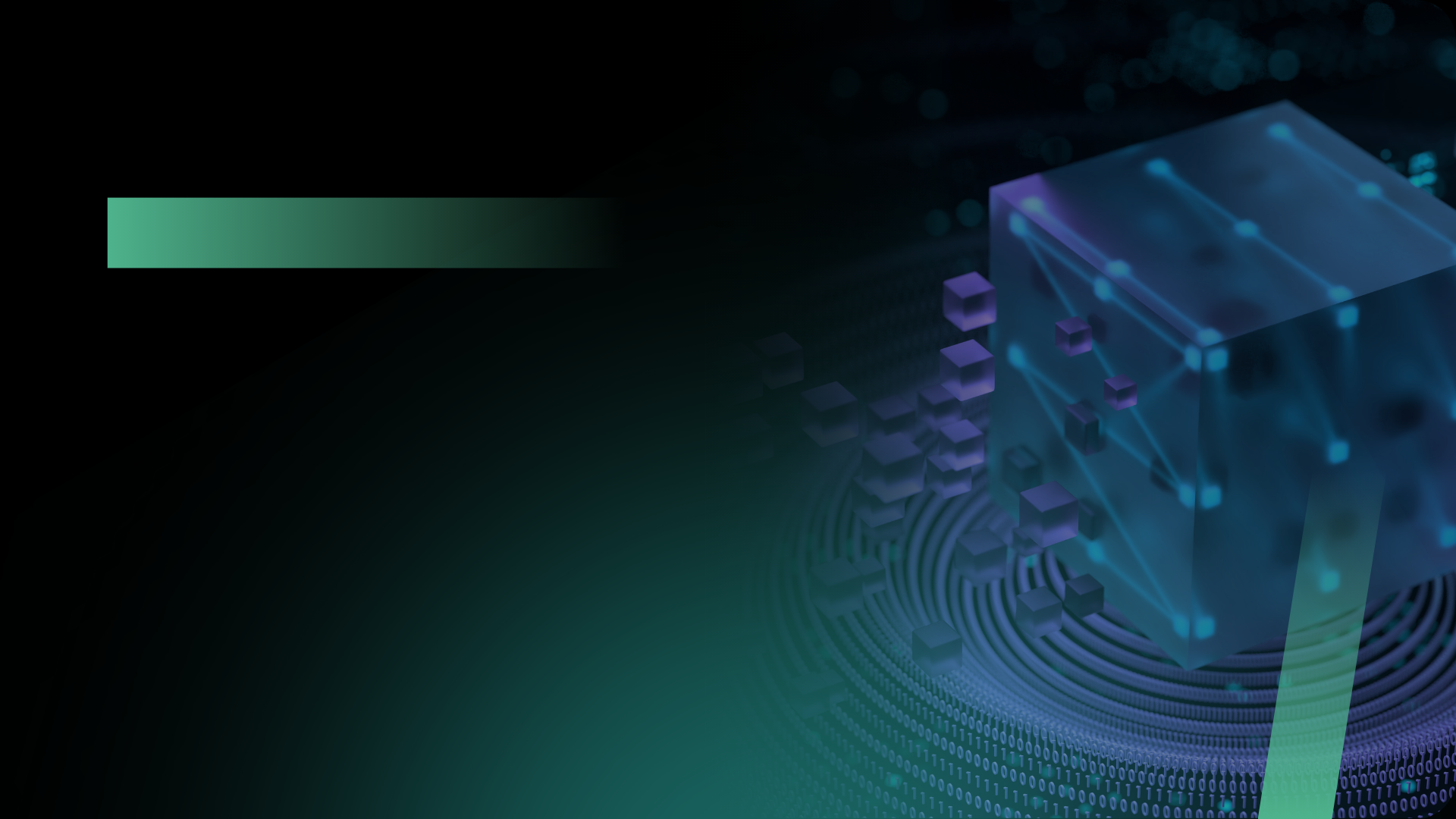
As AI adoption and customer IoT data become more available, data volumes continue to grow. But where do businesses store all this data efficiently until it’s ready for use?
Data lakes have become a cost-effective and smart solution for big data storage, as well as a way to combat the challenges previously faced by organisations when it comes to data storage.
Traditionally, businesses have faced several hurdles to getting the most from their data:
- They didn’t have the time or expertise to clean and filter data before using it.
- They didn’t have the storage space to save everything.
- They couldn’t use advanced analytics to learn valuable insights from the data.
Data lakes help solve these issues.
A data lake is a massive storage solution that allows businesses to save, manage, and analyse vast amounts of data. With so many data pipelines constantly bringing new information, the significance of data lakes has grown. A modern data lake can accommodate millions of gigabytes of various data types, from structured information to unstructured social media, video, and text content.
At their most basic, data lakes address the challenge of storing and using these large data volumes. They provide a scalable and cost-effective platform that simplifies data analysis, making it easier for businesses to derive value and make data platform-driven decisions.
This guide will explore the functionality, importance and impact of data lakes in modern data management. First, it’s important to understand the types of data sources businesses might have.
Data lake use cases
With their ability to store and manage big data, data lakes have become pivotal in modern IT strategies. Below are three key use cases that highlight their utility:
- Data lakes power sophisticated analytics and machine learning projects. They provide extensive, varied datasets for developing and refining predictive models. These bring insights that drive innovation and efficiency.
- Data lakes are an excellent choice for industries where immediate data analysis is crucial. For instance, financial sector fraud detection or manufacturing monitoring IoT devices. The ability to ingest and process streaming data helps make instant decisions in time-sensitive environments.
- Data lakes act as central hubs for diverse datasets. By providing a unified single source of truth, data lakes enable more informed, strategic business decisions across various departments.
What are the different types of data storage?
Understanding data storage is crucial to navigating data management. Each type is distinct yet integral to the whole system.
Structured data
Structured data is as orderly as a grid in a spreadsheet. It comprises data that's systematically arranged in rows and columns, ready for data analysts to pore over. This is typical in relational databases, for example.
In data lakes, this well-organised data integrates seamlessly, enabling streamlined processing and analysis. However, arranging data into a structured format is often extremely time-consuming and challenging.
Unstructured data
Unstructured data, on the other hand, can be varying masses of many data types. It can include everything from email text and videos to social media posts. This data, lacking a conventional structure, is traditionally complicated to gain insights from. But nowadays, it is vital for comprehensive analysis of many aspects of business.
We can now use unstructured data with varying methods and algorithms to obtain previously unseeable information. Within data lakes, it's stored in its native format, ready for more in-depth analytics.
Semi-structured data
Semi-structured data is a mixed data type, combining aspects of both structured and unstructured data. It possesses some organisational features — like XML and JSON files with structured metadata and raw text — yet retains flexibility. Data lakes easily accommodate this hybrid information, enhancing its scope and versatility.
How a data lake works
A data lake is a vast repository for all data types. They’re designed to ingest and store structured and unstructured data in their original form.
This flexibility is the core strength of data lakes.
They allow businesses to store new information without structuring or formatting first. But most crucially, businesses can also learn from their vast volumes of data.
By tapping into raw data, they unveil insights about trends and customer behaviours that might otherwise remain hidden. This direct access to unfiltered data is invaluable for understanding the depths of their business intelligence source material.
As such, data lakes are now primarily used as a hub for big data analytics. They are ideal for applications demanding extensive data volumes and sophisticated analytics, such as data mining, automation and predictive modelling. With a centralised data platform, analysts can quickly locate, prepare, and analyse relevant data. Without such a system, the process becomes much more complex.
Data lake architecture
Traditionally, data lakes have been associated with Hadoop file systems or massive on-premise storage. Nowadays, there's a shift towards building on cloud-based object storage services, which offer easier scalability and flexibility.
Some businesses will simply store anything and everything within their data lake. Some will do initial filtering, data integration or structuring first. Others will create sandboxes and dashboards within their lake for data and business analysts to work on.
No matter its purpose, a data lake requires more than just the right technologies. With such large volumes of information, key elements include a standardised dataset folder structure and best practices. There should also be a method of identifying data quality issues coupled with strong access policies.
Of course, ensuring data security is vital. Measures like data encryption and automated monitoring all contribute to secure storage. All staff should know how to protect their data and minimise cyber security concerns.
Data lakes vs data warehouses — the key differences
Data lakes and data warehouses are fundamental concepts often mentioned in the same setting. Understanding the difference is essential for any business to harness its data effectively.
The contrasts between a data lake and a data warehouse lie in the data types, processing methods, intended users and business objectives:
- A data lake is a vast store of raw data. The nature of this data is not always established – it might just be saved for later. This makes it potentially confusing but offers flexibility and a broad scope for future use. The vast amounts of unstructured or semi-structured data make data lakes a goldmine of potential insights for data scientists.
- In contrast, a data warehouse is a carefully curated data collection. It’s an organised repository that stores structured, processed data ready for analytical purposes using techniques like SQL. They are a more streamlined, structured environment suitable for businesses focused on operational reporting and specific, purpose-driven analytics.
As organisations evolve, the need to work with different data grows. Data warehouses can be slow and difficult to use with relentless streams of unstructured information. This is where data lakes excel, providing the agility and capacity to accommodate a broader range of data formats.
The pros and cons of data lakes
Data lakes offer a modern approach to data storage, providing significant benefits for businesses aiming to leverage their data assets. Key advantages include:
- Flexibility: Data lakes are open format and can be built on private infrastructure or purchased from cloud storage providers.
- Less work: Unlike structured systems, data lakes allow for postponing things like schema design and data preparation until there's a clear business requirement.
- Cost-effectiveness: Building a data lake is a relatively affordable way to store huge amounts of data.
- Scalability: Cloud data lakes excel in scaling up and down as needed while maintaining data integrity, even when saving petabytes of information.
- Advanced analytics: Data lakes support various on-demand analytics methods through modern data platforms.
- Data integration: All enterprise data sources can be collected into one centralised place.
Challenges of data lakes
Despite their benefits, data lakes present several challenges that organisations must consider:
- Complexity: Data lakes can be complicated to deploy, requiring careful selection and integration to meet business needs.
- Data governance: Storing raw data in its original form demands strong data governance to avoid data quality and consistency issues.
- Silos: Without careful planning, data lakes can become fragmented. This can lead to data redundancy and inconsistency.
- Quality: As the name implies, a data swamp is a storage solution that has become unmanageable. Nobody knows what information is inside, and it has little potential to provide useful insights. One of the key considerations for businesses is not allowing their data lake to become a data swamp. To do this, proper management is required to avoid poor quality data, a lack of data governance, and a lack of data cataloguing. If you’re in danger of a data swamp, data governance policies and procedures are your first port of call.
- Expertise: Implementing and managing a data lake requires specialised knowledge, which can be a barrier for some organisations. Working with a third-party cloud solutions partner is often advisable in this case.
How Nasstar can help
From real-time business intelligence to complex data science, data lakes offer a versatile and comprehensive solution for managing and leveraging data. They provide a low-cost way to bring data under one roof, uncovering never-before-seen insights.
Nasstar’s team of experts can help you design and build data platforms that bring your information sources together. With years of experience deploying transformative AWS and Microsoft Azure data lakes, we’ll help you unlock a wealth of actionable business insights.
Speak to a specialist to learn more.
Frequently Asked Questions (FAQs)
Can a data lake replace a data warehouse?
Data lakes and data warehouses offer different use cases and advantages. While data lakes store raw, unprocessed data, data warehouses contain structured, clean data. A data lake isn't a direct replacement for a data warehouse but can complement it, offering simpler data storage and processing.
Can a data lake store structured data?
Data lakes can store both structured and unstructured data. They are highly versatile, holding everything from database-ready structured data to unstructured text, IoT, and social media content. This makes data lakes a comprehensive solution for businesses with varied data storage needs.
Why is a data lake essential for big data analysis?
Data lakes are vital for big data analysis as they can store vast volumes of different data. This opens up analytics and machine learning, providing the raw data to uncover trends and support data-driven business decisions.
Where is a data lake stored?
Data lakes are now commonly stored in cloud-based storage systems for their scalability and flexibility. Cloud-based data lakes are also increasingly popular due to their cost-effectiveness and easy accessibility. However, they can also be hosted on-premise if needed.
What is a data lakehouse?
The emerging concept of a 'data lakehouse' aims to merge the extensive storage capabilities of data lakes with the structured management of data warehouses. This hybrid approach is gaining traction as businesses seek to leverage the best of both worlds.