What are big data solutions and why are they important?
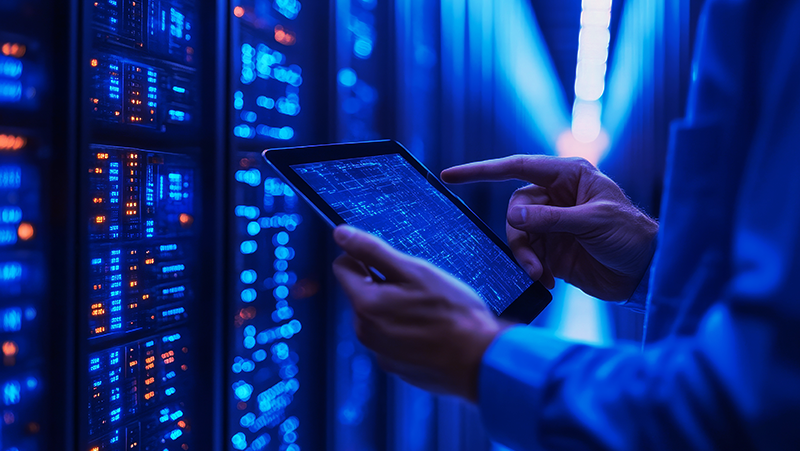
This blog has been expertly reviewed by Andrea Rosales, Lead Data Scientist at Colibri Digital.
We create over 70 times more data per year than in 2010. Between social media, cloud technologies and Internet of Things (IoT) devices, traditional data infrastructure is no longer enough. To manage the vast quantities of information now generated, we’ve turned towards big data solutions.
However, big data's a pretty broad term that has come to describe any information produced in massive quantities. Today, a lot of companies generate and collect information on a ‘big data’ scale – but it doesn’t come cheap. If businesses are going to see a return on investment, all that data must be processed, stored, and analysed appropriately.
Andrea Rosales, Data Scientist at Colibri Digital, said: “Big data is not just about volume; it's about turning massive amounts of information into actionable insights, and unlocking the potential of AI and deep learning.”
In this blog, we’ll learn more about big data, when it’s useful, and which industries stand to benefit the most from its massive potential impacts.
What is big data?
Big data refers to massive, complex datasets created by modern businesses that traditional data processing tools cannot handle. It plays an increasingly important role in strategic decision-making and product innovation. For example, big data insights can help to optimise workflows and uncover new revenue streams and marketing opportunities.
Big data is instrumental in many sectors — from ecommerce to healthcare and finance. It’s capable of giving us deeper insights than have ever been possible. From sentiment analysis and trend prediction to automation, big data solutions help to make sense of the world around us.
The three characteristics of big data
Big data is characterised by the three Vs - volume, velocity and variety:
- Volume: Big data deals with immense datasets. Data storage ranges from terabytes to petabytes through things like social media posts and IoT readings.
- Velocity: This refers to the speed at which data is generated. It’s often processed in real time, which is crucial for timely decision-making.
- Variety: There are hugely diverse forms of data, including structured, unstructured, and semi-structured data formats like text, images and videos.
Advantages of using big data solutions
With proper planning and infrastructure, there are many valuable benefits to using big data solutions.
Andrea commented: “Big data solutions transform decision-making, boost operational efficiency, fuel innovation, and offer a competitive edge by turning insights into strategic actions.”
Enhanced decision making
The first is enhanced decision-making. Big data solutions provide invaluable data-driven insights, allowing businesses to learn actionable information. This supports strategic planning and decision-making by ensuring all choices are backed by evidence.
Predictive analytics, in particular, are a key component of big data. They enable businesses to forecast future trends and behaviours based on previous data, allowing for proactive measures in the future.
Operational efficiency
Streamlining current workloads is another significant advantage of big data solutions. By automating processes, big data enhances efficiency by reducing the need for manual intervention while minimising errors.
Likewise, real-time data monitoring and adjustments mean that business metrics are continuously tracked. Companies can immediately respond to any operational changes, resulting in smoother workflows and enhanced productivity.
Innovation
Large datasets can help organisations identify market gaps and explore potential growth areas. This opens up huge product and service innovation, all from big data insights. Companies can develop new offerings and improve existing ones based on customer feedback and other key metrics.
Competitive advantage
By helping businesses understand market trends and capitalise on emerging opportunities, big data provides a significant advantage over less agile competition.
Companies using big data can personalise customer experiences, tailor products and services, and enhance customer satisfaction, creating a stronger business in the long term.
Types of big data solutions
So, how do companies manage and use their information? From data centres to advanced analytics platforms, there are several key types of big data solutions that each contribute to a thriving business ecosystem.
- Big data analytics: Big data analytics solutions uncover patterns and insights from enormous datasets. Advanced analytics helps identify trends, while predictive analytics forecasts future events. But each plays a key role in enabling modern businesses to make informed decisions based on their data.
- Data storage: Scalability and cost-effectiveness are vital for reliable data platforms. Modern data lakes and data warehouses offer scalable solutions for structured and unstructured data needs. NoSQL databases are often used for unstructured data, providing flexible schema design and high performance. Simpler datasets may still benefit from SQL-based relational databases.
- Data processing: Open source Apache Hadoop has been crucial for big data storage and processing since its initial release in 2006. Other data applications like Apache Spark, known for its fast in-memory processing, support real-time data analytics.
- Data analysis: Business intelligence (BI) tools enable data visualisation. Likewise, machine learning algorithms, used for predictive modelling and data classification, provide valuable knowledge across various industries.
- Cloud computing: Big data requires massive processing power. Cloud-based storage solutions, from providers like Amazon Web Services and Microsoft Azure, offer almost unlimited flexibility and scalability. This frees companies from the limitations of on-premise infrastructure, providing cost-effective, on-demand data management.
Challenges in implementing big data solutions
While big data can bring many benefits, there are also potential challenges to consider. First, ensuring data protection is vital. Robust security measures, including encryption and access control mechanisms, must protect sensitive information. Likewise, compliance with regulations like GDPR is essential.
Reliable analysis also relies on high-quality data. Data cleaning and preprocessing transform raw data into usable formats, employing various techniques to enhance quality.
Scaling can also be tricky. Maintaining performance as data increases presents challenges and often requires new and more robust solutions. Infrastructure costs must be balanced against the anticipated benefits. Working with an experienced cloud solution provider can help you overcome these challenges.
Big data solutions use cases
Many industries can benefit from using big data solutions, including:
- Retail: Big data enhances retail business intelligence. Real-time monitoring of performance allows companies to make fast and informed decisions. In marketing, segmentation and behaviour prediction enable targeted strategies and improved customer satisfaction.
- Healthcare: In the healthcare sector, big data opens up patient data management and predictive diagnostics. These can help identify disease patterns and potential health risks, enabling personalised treatment plans.
- Finance: Big data is crucial in financial services for fraud detection and risk management. Analysing transaction patterns helps identify and prevent fraudulent activities. Predictive models assess and mitigate financial risks, providing a safer and more stable financial environment.
Andrea said: “When choosing a big data solution, we align technical capabilities with business goals, ensure the infrastructure is scalable, and utilise advanced analytics for optimal decision-making.”
How do I choose a big data solution?
Choosing the right big data solution for your business comes in five steps.
1. Assess business needs
Start by understanding your data requirements. It’s important to identify the types of data your business generates and determine their volume, velocity, and variety. Your big data strategy should align with your business objectives by defining key metrics that will tell you if a new project has been a success.
Next, consider your budget constraints. Take a look at the available budget and prioritise big data investments based on the expected return on investment. Then, you can include the total cost of ownership, such as maintenance and operational expenses. Once you have this information, you can judge whether a big data solution will be worthwhile for your business.
2. Plan and identify data sources
Step 2 is where technical planning begins. You can compare the features and capabilities of different big data tools to find those that best meet your business needs. Consult experts and consider multiple different solutions.
It’s important that the solutions are scalable and flexible enough to grow with your data needs. They should adapt to your changing business requirements, not vice versa. Log all internal data sources within your organisation and identify external data sources that could help take your analytics even deeper.
3. Build and manage big data infrastructure
Next, select the right big data technologies for storage, processing, and analysis. Consider things like Hadoop, Spark, and NoSQL databases, depending on your data integration and analytics needs.
At this point, you’ll make an important decision regarding where to place your infrastructure. Some companies prefer the control of an on-premise solution, although this is costly and difficult to build and maintain. Many others prefer the scalability and cost-effectiveness of the cloud.
4. Analyse and visualise data for insights
Once you’ve planned and built your infrastructure, you’re ready to see the results. Techniques like machine learning algorithms can give you deeper analysis to uncover hidden patterns and trends within your data.
You can also use tools for data visualisation, building dashboards with interactive visualisations and key metrics in real time. These are an excellent way to get stakeholder buy-in, providing faster insights and enabling more informed decision-making.
5. Continuous optimisation
Finally, big data solutions are a constant work in progress. To produce results now and in the future, you should monitor your KPIs and tweak things when necessary. You might:
- Monitor performance regularly to optimise workflows
- Implement automated data pipelines and APIs to reduce manual efforts and improve accuracy
- Get technical support from your partners
- Provide training for staff on your big data tools and technologies
How Nasstar and Colibri Digital can help
Big data solutions help businesses make sense of their huge volumes of information. With data generation coming from all angles, including customer feedback, online sources and IoT devices, companies can learn more than ever. All it takes is the right expertise and infrastructure.
At Nasstar and Colibri Digital, we can help you build effective, reliable and scalable big data solutions. Whatever stage of your data project you’re at, our expert team has the knowledge to help you make it a success. Speak to a specialist to learn more.
Frequently asked questions (FAQs)
What is big data with examples?
Big data means the massive amount of information that modern companies generate and store. It can be used in many ways, including customer service, new product creation, and improving business efficiency.
What is an example of a big data platform?
A popular example of a big data platform is Apache Hadoop. Released in 2006, it is an open-source platform that allows for the processing and distribution of vast quantities of data.
What is big data being used to solve?
Big data is being used to solve many important issues. For example, the healthcare industry is using big data to uncover new ways to diagnose diseases. Meanwhile, it is also increasingly used in predictive medicine.
How do you implement a big data solution?
To implement a big data solution effectively, it’s essential to follow a well-planned outline:
- Understand your business, its aims and budget
- Get an overview of your data and data sources
- Build an infrastructure that matches your needs
- Conduct your data analysis
- Continually work to improve your system